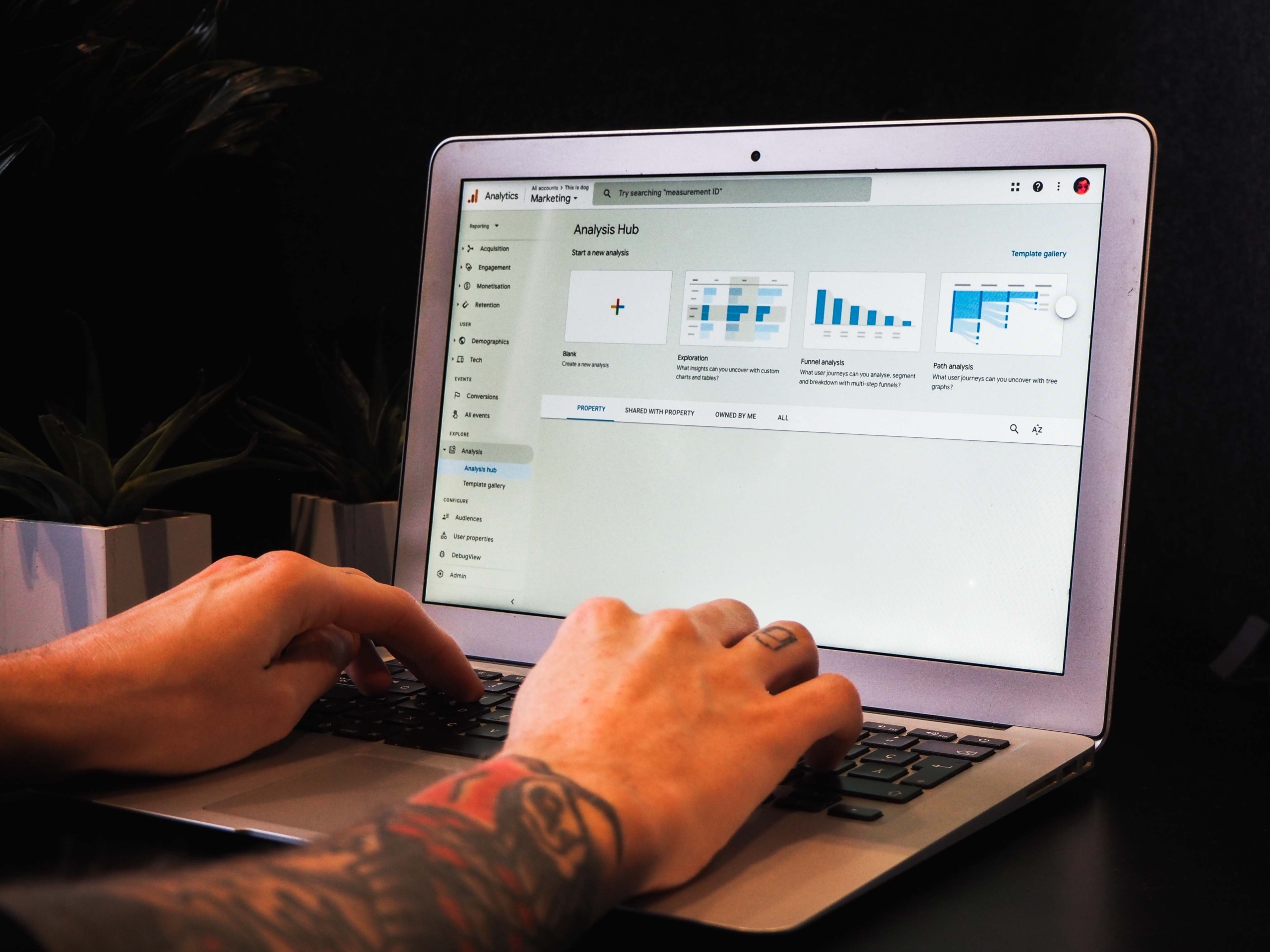
Author: Christopher S. Penn, Co-founder & Chief Data Scientist at Trust Insights
Predictive analytics is a discipline that’s been around in some form since the dawn of measurement. We’ve always been trying to predict the future; go back in history to look at prognosticators like Nostradamus and many other prophets. Predictive analytics have been practiced since the first line graph was drawn and someone put a ruler on the chart to ballpark the trend happening in their business.
A Brief History of Predictive Analytics
No industry has attempted to do more with predictive analytics than the financial services industry. From the opening of Lloyd’s Coffee House in 1686, financial services professionals have been attempting to forecast what’s going to happen next. Financial Planners, CFOs, and analysts have all struggled to build accurate methods for predicting what’s likely to happen.
Prior to the dawn of advanced statistical analysis and machine learning, predictive analytics efforts fell into 4 broad categories:
1. Guessing, which is the default that most people revert to. Based on qualitative and quantitative data plus subject matter expertise, folks will attempt to guess what’s likely to happen. Whether or not the results of such forecasts beat random chance is highly dependent on the subject matter expert’s skills.
2. Visual forecasting, which is a polite way of saying get out a ruler and draw lines on paper. This technique has been popular for decades and for good reason; for data where you have regular linear patterns, drawing lines on charts works. You’ll see this still today with things like stock analysis, particular in broadcast media. Analysts will draw lines for the floor and ceiling prices of stocks to infer whether or not it’s worth buying those stocks.
3. Additive forecasting, a popular method of doing budgeting in which you take the previous period’s data, adjust it by a percentage, and then call that your forecast. This assumes that the upcoming period will be identical to the previous period in terms of forecast, which works only under certain conditions. In an era with a pandemic and political instability, it’s not a sound strategy unless it comes with exceptional agility and nimbleness to change when circumstances change.
4. Making things up, which is the least useful method of forecasting but one that a surprising number of organizations and stakeholders do in the absence of any other forecasting techniques.
The first three techniques have the same thing in common: they take existing data and attempt to use it to build future-facing models. What’s changed in the last 25 years and especially in the last 10 years is the use of advanced statistical and machine learning software to build forecasts that are mathematically more sound than these early approaches.
What Predictive Analytics Cannot Forecast
By extension, data which completely lacks seasonality or cyclicality cannot be predicted or forecast. This latter point is critical for understanding the limitations of predictive analytics. There are four general categories of data that you cannot predict with any level of accuracy:
- Things that have never happened
- Things that have no predictability
- Things that have no cyclicality or seasonality
- Things that have hidden inputs
For example, when it comes to things that have never happened, predictive analytics software – no matter how good, how sophisticated, how much data – could not have predicted with any level of accuracy the COVID-19 pandemic. Nor could they have successfully predicted any number of the “black swan” events in any reasonable fashion; while some nations’ military intelligence capabilities might have detected these threats sooner rather than later, for the vast majority of businesses and finance departments, these are truly unique events.
Predictive analytics, by definition, cannot predict what is unpredictable. A subtle example of this is political elections, such as the United States presidential election. While these elections occur every four years like clockwork, the reality is that no two elections are ever alike. The candidates change, the issues change, and even the voting base changes substantially between elections. Thus, no predictive model works to predict elections, despite the best efforts of many to the contrary.
Predictive analytics cannot predict randomness, things which have neither seasonality nor cyclicality. For example, suppose you’re working with a video production team to publish videos on YouTube explaining a highly complex subject. By chance, one of those videos does really well and takes off. There’s no way to predict that sort of randomness, even though your production schedule is very predictable.
Finally, predictive analytics cannot forecast massively complex models with hidden inputs. The stock market is an excellent example of this. Enormous resources have been invested in attempting to predict the stock market’s performance in aggregate as well as in individual equities, but there are so many hidden inputs, from HFT algorithms to macroeconomic events that models simply cannot predict with accuracy much better than guessing.
Predictive Analytics Example in Finance
Let’s look at a very specific example of predictive analytics, something that my company Trust Insights did with a client years ago, in the casino industry. We worked with the Foxwoods Casino in Connecticut, USA who asked us to help use finance data to create a forecast. Casino attendance and casino receipts are highly predictable. There’s clear cyclicality and seasonality in the data, making it a prime candidate for forecasting.
We examined three years of casino receipts and using custom-built machine learning software, we forecast their projected revenues, week-by-week, for 52 weeks ahead. With that forecast, they could easily determine what weeks in the coming year were likely to have lower revenues than others.
The key with predictive analytics, like all analytics, is not the forecast itself but what you do with it, and this is where the forecast really found its power. With the knowledge of specific weeks that revenue was anticipated to be down, Foxwoods engaged its marketing teams to create promotions around those weeks, helping to drive additional foot traffic and offset those weak periods. These involved campaigns like local press tours, digital advertising, rewards to existing members, special promotions and pricing – all the tools they had in their toolbox to attract and retain customers.
The net effect? Instead of a down year compared to previous years and what was originally forecast, Foxwoods saw 29% year-over-year revenue growth, an astonishing number. What’s really interesting was that the following year, with a change in management and a change in marketing agencies, Foxwoods opted not to engage our team and their revenues were down to the baseline from the year before – in other words, a 29% drop year-over-year in revenue.
Again, the critical point here isn’t the forecast, but what we do with the information – the actions we take, the decisions we make. Predictive analytics is like all analytics – if we do nothing with the data, it’s a distraction at best. Only when we make decisions based on the analysis do we unlock the value of predictive analytics.
This article as it was published on the Jedox website: https://www.jedox.com/en/blog/predictive-analytics/